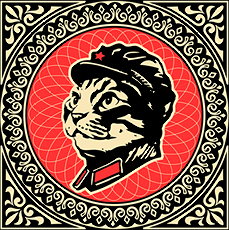
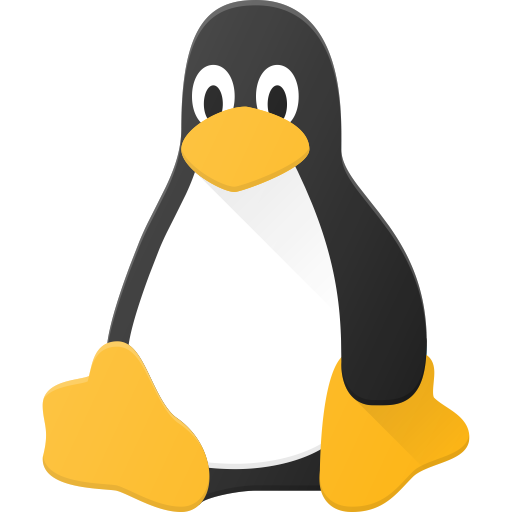
Except the part where it said downloading videos is against their terms of service? Which was my only point?
Did you completely fail to read the part “except where authorized”? That bit of legalese is a blanket “you can’t use this software in a way we don’t want to”.
You physically cannot download files to a browser. A browser is a piece of software. It does not allow you to download anything
Ah, you just have zero clue what you’re talking about, but you think you do. I can point out exactly where you are on the Dunning-Kruger curve.
This is such a wild conversation and ridiculous mental gymnastics. I think we’re done here.
Hilarious coming from you, who has ignored every bit of information people have thrown at you to get you to understand. But agreed, this is not going anywhere.
I’m surprised to hear GIMP crashed on you, I don’t think I’ve ever had it crash on me.